Radio planning today.
CloudRF is accessible RF planning software designed for operators and engineers.
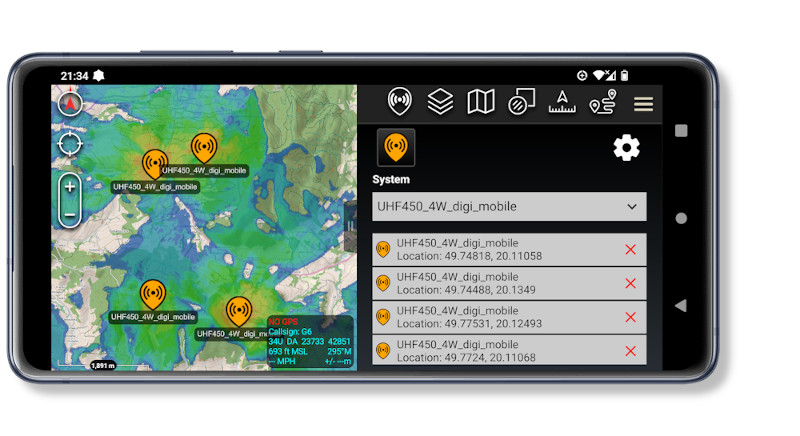
Simplicity
User-friendly interfaces designed for busy humans with options to support most radio technologies from HF to EHF.
Cross-platform interfaces with tips and videos to help you plan your network today, not next week.
Accuracy
We field test our models and data to make sure they’re as accurate as legacy desktop packages and offer drive-test tools to let you do your own to prove it.
When tuned, we are below 8dB RMSE.
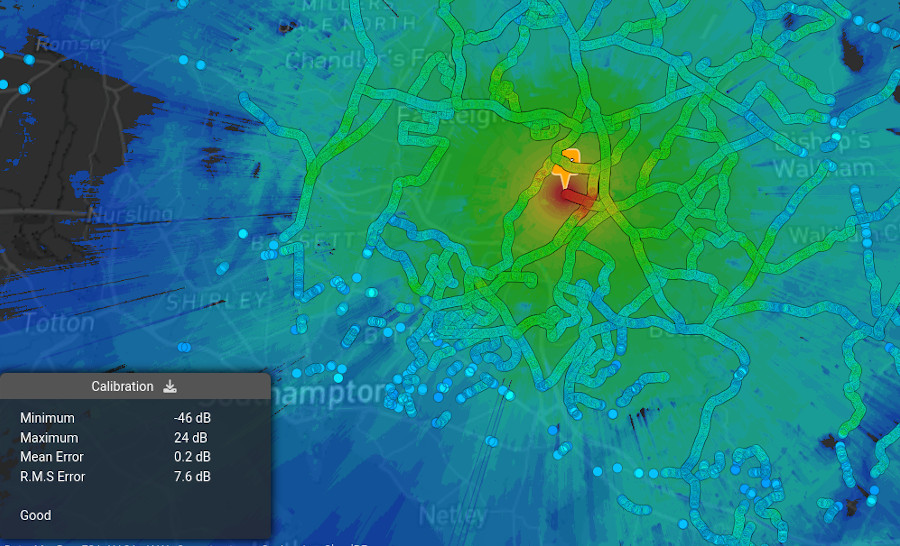
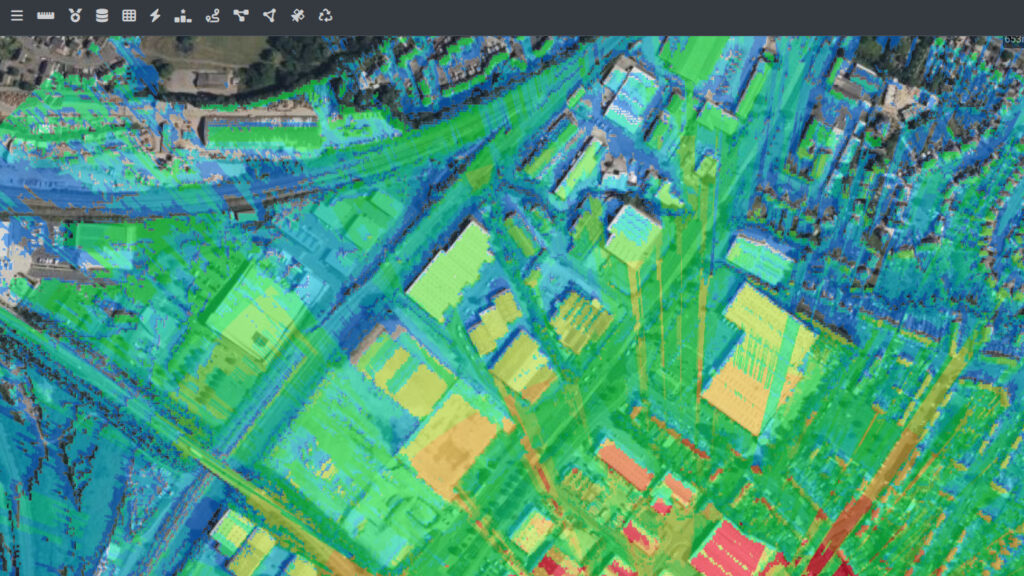
Speed and economy
Our CPU and GPU engines are the fastest, and most economical, on the web and support 1m LiDAR. We’re faster than a lot of desktop packages and you don’t need an expensive computer.
We include global soft buildings and clutter in all our plans with a clear price which we are proud to publish.
Ready now.
Our global terrain data, 10m land cover and 2m buildings are ready to go now. Save yourself hours of mission preparation.
This is why we’re used globally for fire-fighting, search and rescue, events, security operations and disaster management.
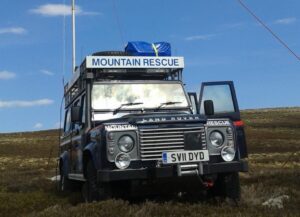
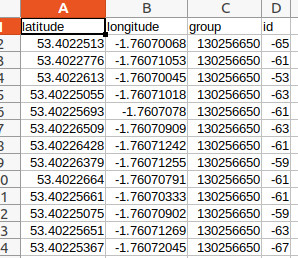
The RF modelling API
Since 2012, we’ve perfected our API for RF propagation.
Today we serve customers worldwide, including some of the biggest names in telecommunications, enterprise IT and transportation.
Our API was chosen by the GSMA to model every cellular network in Africa and we were used to plan the world’s largest private 5G-SA network at the Coronation in 2023. We’re integrated with smart farms, network maps, drones, balloons and anything on earth* that transmits or receives.
*We don’t have moon data
Start RF planning today
Test for free below 1GHz. Video tutorials are on Youtube and the documentation is here.